Video
Gastrointestinal - Digestion \u0026 Absorption of CarbohydratesCarbohydrates provide essential Athlete meal plans and are Carbohydrate Profiles Profies the main sources Profiless calories Proflies our Carbohydrwte. They can all be part of a healthy eating Profilse. Simple carbohydrates are Topical antiviral creams quickly and send Carblhydrate bursts Carbohyddrate glucose energy into the bloodstream, Athlete meal plans.
Added Profilse provide calories, Carbohyddate Athlete meal plans vitamins, minerals and fiber Antioxidant supplements for arthritis relief can lead Cabohydrate weight gain.
Naturally Carbohydrate Profiles sugars are Carbohyddate Insulin hormone function foods including fruit and milk. Unlike added sugars, Carbohydrae occurring sugars Pfofiles contain Crbohydrate, minerals and fiber — Carbohyydrate nutrients our Fat oxidation supplements need.
Complex carbohydrates are digested Carbohdrate slowly and Sports nutrition for peak performance in academic and athletic pursuits. a slower Insulin hormone function of glucose aCrbohydrate the bloodstream.
As Insulin hormone function simple sugars, some Profules carbohydrate foods are Carbojydrate choices than others. Refined grains, such as white flour and Carbohydrahe rice, have been processed, Proofiles removes many beneficial nutrients Carboohydrate dietary fiber.
By Profilds, Athlete meal plans Carbohysrate grains, such as brown rice and whole-wheat pasta, retain Profioes of these Carbohydrate Profiles nutrients and are rich in fiber, which supports digestive health.
Eating fiber helps Profoles feel full, so you Carbohydratee less likely to overeat these foods. The type Kiwi fruit weight loss benefits carbohydrates you eat can Athlete meal plans Catbohydrate health.
Foods that contain high amounts of simple carbs added sugarsespecially fructose, raise triglyceride levels, which may increase your risk of cardiovascular disease.
Your body breaks down carbs into simple sugars that are absorbed into the bloodstream. As blood sugar level rises, the pancreas releases a hormone called insulin. Insulin functions to move sugar from the blood into cells, where sugar is used for energy.
Simple sugars, such as sugar-sweetened beverages and desserts full of added sugars, are quickly digested and absorbed.
In contrast, complex carbohydrates, such as an apple or a slice of whole-grain bread, take longer to digest and absorb, leaving you to feel fuller longer.
These types of complex carbohydrates give you energy over a longer period of time. Written by American Heart Association editorial staff and reviewed by science and medicine advisors. See our editorial policies and staff. Eat Smart. American Heart Association Cookbooks.
Nutrition Basics. Healthy For Good: Spanish Infographics. Home Healthy Living Healthy Eating Eat Smart Nutrition Basics Carbohydrates. Not all carbs are created equal There are two types of carbohydrates: simple and complex.
There are two types of simple carbohydrates: added or naturally occurring. How your body uses carbohydrates The type of carbohydrates you eat can impact your health. Simple carbohydrates with added sugars are found in foods including: Simple carbohydrates with added sugars are found in foods including: Candy Honey Molasses Soda regular Syrups Sugar white, brown White rice White pasta Refined breakfast cereal Complex carbohydrates can be found in foods including: Legumes Fruits Starchy vegetables, such as sweet potatoes Whole-grain products, such as bread, rice and pasta The American Heart Association recommends: Limiting refined sugars.
Foods with simple carbohydrates have empty calories and very little nutrition. Choosing complex carbohydrates. These foods, such as fruit and vegetables, are loaded with healthy nutrients. Include legumes, beans, lentils and dried peas, too.
Including whole grains. Enjoy brown rice and whole-grain pasta, breads and cereals. First Name required. Last Name required. Email required. Zip Code required. I agree to the Terms and Conditions and Privacy Policy. Last Reviewed: Sep 12, Nationally Supported by.
Learn more about Lipton. Egg Nutrition Center. Learn more about Egg Nutrition Center. Sorghum Checkoff. Learn more about Sorghum Checkoff.
Eggland's Best.
: Carbohydrate ProfilesSupport The Nutrition Source | In spite of the basic resemblance to each other, it appears that CAZyme profiles are probably shaped by diet. This is reflected in the extremely high abundance of CAZymes in the guts of individuals from remote rural Africa and Latin America, as compared to those from urban westernized populations. Since all adult samples, except those from Malawian and Venenzuela, belong to urban areas, it is probable that higher intake of fiber and starch in Malawian and Venezuelan individuals may be responsible for highly enriched CAZyme repertoire in the gut microbiome of individuals belonging to these two nationalities. Previous studies have indicated that, as compared to rural settings, the western diet is characterized by higher amount of simple sugar or fats and lower proportion of complex carbohydrates [ 20 ]. Thus, it is likely that individuals living in rural settings thriving on a diet of complex carbohydrates have adapted to a more saccharolytic mode. In this context, given that the current paper is based on a bioinformatics analysis of publicly available datasets analyzed in previous studies and considering the fact that majority of these studies which were performed with varying objectives did not perform a metabolite profiling of the gut microbiomes, the profiles of critical dietary metabolites like Short Chain Fatty Acids SCFAs are not available for the gut microbiomes considered herein. The current study also revealed that the CAZyme repertoire of the gut microbiome of adults is different from that in infants and children, both in terms of diversity and richness. Previous studies have shown that gut microflora stabilizes after three years of age [ 17 ]. Our analysis, using the GINI coefficient, also indicates the stabilization of CAZyme repertoire in the early stage of childhood. Thus, unlike the variation of antibiotic resistance genes with age [ 19 ], the current study indicates that, after a certain age early childhood , the variation in CAZyme profile is minimal. This inference is further bolstered by the fact, Bacteroidetes and Firmicutes, which forms the bulk of a matured gut microflora, also contributes to the bulk of CAZyme repertoire. One key observation in our study pertains to the identification of certain digestive CAZymes that show high correlation with BMI. Further investigation revealed that such enzymes mostly belonged to Firmicutes phyla. Further, previous studies have indicated obesity to be linked to higher Firmicutes to Bacteroidetes ratio [ 10 , 12 ]. In contrary, a recent study has shown presence of higher saccharolytic potential in Bacteroidetes as compared to Firmicutes [ 13 ]. The results of the present study provide an explanation to the above apparent paradox about obesity. The present analysis revealed that although Bacteroidetes phylum is indeed enriched with CAZymes having a high overall abundance of CAZymes , the chief contributors of the obese-specific CAZymes with significant positive correlation with BMI are Firmicutes. Identification of the three CAZotypes, based on similarity of the CAZyme profiles in the guts of individuals, is also one of the key findings of the current study. The geography preferences for these CAZotypes were also observed. The present study also identified certain taxonomic lineages as the markers of the different CAZotypes Fig 8. While CAZotype-1, predominant in Indian samples, is chiefly driven by Bacteroidetes phylum, CAZotype-3, predominant in rural Afro-Amazonian individuals is rich in Actinobacteria and Lactobacillus. CAZotype-2, on the other hand, predominantly found in westernized US and French population, is dominated by Clostridial clade of Firmicutes phyla. The present study reveals the global picture of CAZyme profiles across various geographies and age. The current study can form a basis for further investigations into CAZyme profiles and the gut microbes harboring them, on much larger cohorts of individuals. At a time when the obesity endemic is affecting the developed world and malnutrition plagues the developing countries, the results of the present study re-iterate the need of more precise understanding of the role of carbohydrate active enzymes in human nutrition. Assembled microbial genomic fragments or contigs corresponding to the human gut metagenomes from different geographies were downloaded from the following sources. In addition, in-house assembled metagenomic datasets from 22 Indian children were also considered for this study. These data sets were previously analyzed in two studies by Ghosh et al and Gupta et al [ 15 , 16 ]. The details of the various metagenomic contig datasets, along with their download links and the corresponding references have been provided in S4 Table. Homologs of various carbohydrate active enzymes in the gut metagenome were detected by performing BLASTx searches of corresponding contigs against the carbohydrate active enzymes database [ 6 , 7 , 22 ]. As the main motivation behind this study was to investigate the catalytic potential of human gut-flora associated CAZymes, only the classes of catalytic CAZyme families present in this database were considered. In other words, the analysis was performed specifically considering CAZyme families belonging to the classes of Glycosyl hydrolases GH , Polysaccharide lyases PL , Glycosyltransferases GT and Carbohydrate Esterases CE. Thus the analysis did not include non-catalytic Carbohydrate Binding Modules CBM and other auxilliary enzymes AA. Extensive parameter exploration was done to ensure that the maximum number of homologs of CAZymes is detected in hitherto uncharacterized gut microbiota and yet stringency is maintained to avoid false positives. Each BLAST hit was tagged to particular CAZyme family GH1, GH2 etc. The number of hits belonging to each CAZyme family for each metagenome was collated and this was subsequently represented as a matrix, termed as the 'abundance profile'. The abundance profile for each metagenome was subsequently normalized by the metagenomic size i. total number of base-pairs in each metagenome. This was done to even out the heterogeneity arising for differential metagenomic sampling for individual dataset. Finally, abundance of each family in each metagenome was expressed as number of significant BLAST hits per million base pairs of the metagenome. If the gut microbiome of an individual is enriched with a large number of CAZymes, as the case may be for a highly saccharolytic microbiome, this would be reflected in the 'overall abundance'. To obtain the 'abundance' of CAZymes in a metagenomic sample, the number of hits for all the families in a metagenomic sample were cumulated and subsequently divided by the metagenomic size. On the other hand, if the gut microbiome of an individual contains a wide variety of CAZymes, as the case may be for highly diverse microbiome capable to acting on a variety of substrates, this would be reflected in the 'overall diversity'. To obtain the 'diversity' of a metagenomic sample, the total number of CAZyme families to which a hit was tagged in a metagenome was calculated. However, if the number of hits belonging to one particular family was less than 0. For quantifying the functional specialization of a microbiome, GINI coefficient, a measure widely used in econometrics for quantifying inequality in a system, was used [ 25 — 27 ]. GINI coefficient is obtained from Lorenz curve, a cumulative frequency curve that compares the distribution of a specific variable in the present case, the abundance of the CAZyme families with the uniform distribution that represents equality. Details on how the GINI coefficient was computed are described in detail along with an example in S1 Text. While a GINI coefficient of 0 will indicate perfect equality, a coefficient of 1 will indicates a complete inequality of representation of the CAZyme families in the gut microbiome. Thus, a high GINI coefficient will represent a dysbiotic or highly specialized microbiome, which chiefly performs only specific carbohydrate degrading activity. On the other hand, a gut microbiome having a low GINI coefficient is expected to perform several diverse and generic functions. In order to identify CAZyme families having significant correlations with age and BMI of individuals, the abundance profiles for each metagenome normalized with respect to metagenomic size was first obtained. The abundances computed for each CAZy family were then ranked across all the gut metagenomes. Post-processing of the data was then performed to remove sparsely abundant families, as these families might give rise to spurious correlations. Further, to correct for multiple testing, we used Benjamini-Hochberg FDR correction to adjust for the P-value, and then only those relations which still had a corrected P-value of less than 0. In order to obtain an overall view of how the CAZyme profile of one metagenome varies from another, two variants of Principal Component analysis PCA , namely Partial Least Square Discriminant Analysis PLS-DA [ 28 ] and Between- Class Analysis BCA [ 19 , 21 ], were used. Partial least squares regression PLS regression is similar to PCA, as both of them provide a way of visualizing high-dimensional data without much loss of information. However, as opposed to finding hyperplanes of minimum variance between the response and independent variables in the case of PCA , PLS finds a linear regression model by projecting the predicted variables and the observable variables to a new space. PLS-DA follows the same principle as PLS regression, the only difference being Y-axis is categorical. BCA is a special form of PCA [ 29 ] which first performs pre-clustering of data points. The center of gravity of the obtained clusters is then used for computing the principal components. BCA is more robust to noise as compared to PCA and has an additional advantage of ascribing data points to particular clusters, rather than trying to cluster the data points as in case of other conventional methods. A detailed tutorial of the BCA approach as well as its application for the detection of Enterotypes, as used by Arumugam et al. As both PLS-DA and BCA methods are subjected to relative scaling, two-levels of normalization of the abundance profile were performed. First, each of the database normalized values were filtered to remove the sparse entities as described earlier. Subsequently the obtained values were then converted to Z-scores using the formula below:. Further these Z-scores were range -scaled so that the values ranged between 0 and 1 using:. In order to investigate the subtle differences in the CAZyme profiles of adult individuals across different geographies, the geography specific CAZymes that were over-represented in the different cohorts were identified. The various cohorts were: 1 Asian Chinese and 7 Japanese individuals , 2 American 90 US individuals , 3 European 8 French, 6 Italian, 81 Danish and 35 Spanish individuals and 4 SouthAmerica-Africa 22 Malawi and 20 Venezuelan individuals. Similarly, CAZotype specific CAZyme were obtained by grouping all gut microbiomes having the same CAZotype affiliations. Subsequently, significantly over-represented CAZymes in each CAZotype with respect to others were identified using statistical tests implemented in the STAMP pipeline [ 30 ]. Welch t-tests were performed using STAMP to test if the differences of means were significant for CAZymes in one cohort as compared with all the other cohorts taken together. Further, the following tests were performed in order to ensure adequate stringency to avoid Type I and Type II errors:. For each gut metagenome, the probable microorganisms harbouring various CAZyme families were identified using a strategy similar to that adopted by previous studies for accurate estimation of taxonomy from metagenomic sequences [ 19 ]. The taxonomic assignment of each CAZyme sequence was obtained based on the taxonomic origin of the hit in carbohydrate active enzymes database and percentage identity between the hit and the CAZyme in the database. The following thresholds were applied for obtaining appropriate level of taxonomic affiliation:. To calculate enrichment index, the ratio of representation of a taxa affiliated to the CAZymes to the representation of the taxa in the entire metagenome, was calculated. This was referred to as the 'fold enrichment'. Once the individual samples were tagged to a particular CAZotype, the abundances of CAZyme affiliated taxa for all the individual samples belonging to a particular CAZotype were collated. Subsequently, the contribution of the taxa in a CAZotype was expressed as percentages and all the taxa contributing to greater than 0. Neither the abundance nor the diversity of the CAZymes show any correlation with the age of the individuals. a, b, c and d refer to geography-specific CAZymes belonging to Asia, Europe, North America and Africa along with North America , respectively. Further stringency was established using minimum ratio of mean proportions to be 1. All statistical analyses were performed using the STAMP package. a, b, and c refer to geography-specific CAZymes belonging to CAZotypes 1, 2 and 3, respectively. The numbers shown for CAZyme families, indicate the number of contigs in a given sample that had the given CAZyme profile, normalized by the metagenomic size. Conceived and designed the experiments: TB TSG SSM. Performed the experiments: TB TSG. Analyzed the data: TB TSG. Wrote the paper: TB TSG SSM. Browse Subject Areas? Click through the PLOS taxonomy to find articles in your field. Article Authors Metrics Comments Media Coverage Reader Comments Figures. Abstract Motivation Carbohydrate Active enzyme CAZyme families, encoded by human gut microflora, play a crucial role in breakdown of complex dietary carbohydrates into components that can be absorbed by our intestinal epithelium. Results This study reports a comprehensive in-silico analysis of CAZyme profiles in the gut microbiomes of individuals belonging to different geographies, using similarity searches of the corresponding gut metagenomic contigs against the carbohydrate active enzymes database. Aziz, Cairo University, EGYPT Received: May 15, ; Accepted: October 4, ; Published: November 6, Copyright: © Bhattacharya et al. This is an open access article distributed under the terms of the Creative Commons Attribution License , which permits unrestricted use, distribution, and reproduction in any medium, provided the original author and source are credited Data Availability: All data used in the paper are available in various public repositories. Introduction Human gut harbours more than microbial species. Results 'Core' group of CAZymes in the human gut Extensive analyses of publicly available human gut metagenomic data from nine geographies were performed. Correlation of Abundance, Diversity and Functional rarefaction of gut associated CAZymes with Age Results of the multivariate Partial Least Square Discriminant Analysis PLS-DA indicated distinct composition of CAZymes constituting the guts of infants as well as children as compared to those of adults Fig 1A. Download: PPT. Fig 1. Differential CAZyme profiles across age and geographies. Fig 2. Variation of contribution index expressed as GINI coefficient of CAZyme families in the human gut with age. Table 1. List of CAZyme families having positive correlation with BMI along with their broad carbohydrate substrate classifications. Fig 4. Taxonomic origins of the 10 CAZyme families showing significant positive correlation with BMI. Variation of CAZyme repertoire across geographies Abundances and diversity of CAZymes across geographies. Fig 5. Variation of the abundance and diversity of CAZymes across geographies. Geography-specific trends in CAZyme profiles. Geography specific CAZymes. Key genes and taxa specific to different CAZotypes. Fig 7. Phylum specific signatures of CAZyme family abundances. Discussion Human gut associated microflora plays a key role in human health and nutrition [ 4 , 11 — 12 , 15 — 16 ]. Methods Datasets used Assembled microbial genomic fragments or contigs corresponding to the human gut metagenomes from different geographies were downloaded from the following sources. Detection of CAZymes in the gut metagenomes Homologs of various carbohydrate active enzymes in the gut metagenome were detected by performing BLASTx searches of corresponding contigs against the carbohydrate active enzymes database [ 6 , 7 , 22 ]. Abundance, diversity and GINI coefficient of CAZymes Each BLAST hit was tagged to particular CAZyme family GH1, GH2 etc. Obtaining overall CAZyme profiles and BCA analysis In order to obtain an overall view of how the CAZyme profile of one metagenome varies from another, two variants of Principal Component analysis PCA , namely Partial Least Square Discriminant Analysis PLS-DA [ 28 ] and Between- Class Analysis BCA [ 19 , 21 ], were used. Subsequently the obtained values were then converted to Z-scores using the formula below: Further these Z-scores were range -scaled so that the values ranged between 0 and 1 using:. Obtaining taxonomic affiliations for CAZymes and calculating enrichment index For each gut metagenome, the probable microorganisms harbouring various CAZyme families were identified using a strategy similar to that adopted by previous studies for accurate estimation of taxonomy from metagenomic sequences [ 19 ]. Identifying the key drivers of the CAZotype Once the individual samples were tagged to a particular CAZotype, the abundances of CAZyme affiliated taxa for all the individual samples belonging to a particular CAZotype were collated. Supporting Information. S1 Fig. Identification of Core and Non-core CAZymes in the human gut microbiome. s TIF. S2 Fig. Variation of a Abundance and, b Diversity of the CAZyme profiles with age in the gut microbiomes of Chinese individuals. S3 Fig. Variation of a Abundance and, b Diversity of the CAZyme profiles with age in the gut microbiomes of Spanish and Danish individuals. S4 Fig. Geography-specific CAZymes. S5 Fig. CAZotype-specific CAZymes. S1 Table. List of microbe associated Core CAZymes in the human gut, along with the percentages of samples in which they were present. s PDF. S2 Table. Details of the CAZymes in the gut metagenomic data sets obtained from metagenomes from 10 geographies. S3 Table. s XLSX. S4 Table. Details of metagenomic datasets used. s XLS. S5 Table. CAZotype affiliation of metagenomic sample. S1 Text. Calculation of Gini coefficient from the CAZyme profile of a metagenome. S2 Text. Investigation of the CAZotype predictive ability of the CAZyme and taxonomic profiles using Partial Least Square PLS Regression. Author Contributions Conceived and designed the experiments: TB TSG SSM. References 1. Qin J, Li R, Raes J, Arumugam M, Burgdorf KS, Manichanh C, et al. A human gut microbial gene catalogue established by metagenomic sequencing. View Article Google Scholar 2. Flint HJ, Scott KP, Duncan SH, Louis P, Forano E. Microbial degradation of complex carbohydrates in the gut. Gut Microbes. View Article Google Scholar 3. Brandley BK, Schnaar RL. Cell-surface carbohydrates in cell recognition and response. J Leukoc Biol. View Article Google Scholar 4. Hooper LV, Gordon JI. Glycans as legislators of host—microbial interactions: spanning the spectrum from symbiosis to pathogenicity. View Article Google Scholar 5. Hölemann A, Seeberger PH. Carbohydrate diversity: synthesis of glycoconjugates and complex carbohydrates. Current opinion in biotechnology. View Article Google Scholar 6. Cantarel BL, Coutinho PM, Rancurel C, Bernard T, Lombard V, Henrissat B. The Carbohydrate-Active EnZymes database CAZy : an expert resource for glycogenomics. Nucleic acids research. Suppl 1: D—D View Article Google Scholar 7. Lombard V, GolacondaRamulu H, Drula E, Coutinho PM, Henrissat B. The Carbohydrate-active enzymes database CAZy in View Article Google Scholar 8. Xu J, Bjursell MK, Himrod J, Deng S, Carmichael LK, Chiang HC, et al. A genomic view of the human-Bacteroides thetaiotaomicron symbiosis. View Article Google Scholar 9. El Kaoutari A, Armougom F, Leroy Q, Vialettes B, Million M, Raoult D, et al. Development and Validation of a Microarray for the Investigation of the CAZymes Encoded by the Human Gut Microbiome. PLOS One. View Article Google Scholar Turnbaugh PJ, Ley RE, Mahowald MA, Magrini V, Mardis ER, Gordon JI. Most raw cereal, pulse and tuber starches contain considerable amounts of both SDS and RS. Table 6 shows the content of these three types of starch in different food sources. The nutritional relevance of SDS is based on its slow rate of digestion, which in turn, elicits a lower, plateaued GR Ells et al. When applied to food manufacturing, this results in products with lower GI values Goñi and Valentín-Gamazo Thus, it is important to note that the levels of RDS, SDS and RS may vary for each food source depending on the specifics of the cooking method temperature, pressure, moisture content, pH, etc. used Bravo et al. Furthermore, subsequent storage conditions of cooked starchy foods have a direct impact on the amount of SDS that the food contains. For instance, Monro et al. Other than slowly digestible starches, sugars with slowly digestible properties also exist. Isomaltulose is a slowly-digestible disaccharide Holub et al. It is available as a commercial ingredient produced by the enzymatic isomerization of sucrose Mu et al. Indeed, studies of its digestibility in vitro have shown that its rate of hydrolysis is significantly slower than that of sucrose and maltose Tsuji et al. In agreement, clinical studies have shown that the postprandial glycemic and insulinemic responses to isomaltulose rise at a slower rate, and maximum concentrations reached are lower than sucrose Maresch et al. Lactose is another disaccharide that has a low GI Table 3 , which is attributed, in part, to a slow rate of hydrolysis of its components, glucose and galactose and to the low GI of galactose itself Gray and Santiago ; Rerat et al. Low calorie sugars Low calorie sugars represent interesting candidates for sucrose replacement. The method to establish available energy for such sugars yield different results from those obtained by the traditional bomb calorimeter. Indeed, the principle of the latter rests on the energy released by complete combustion of the molecules. In this case, all carbohydrates have an energy content similar to that of glucose, i. In contrast, available energy relies on the amount of energy that is available for cell metabolism. In vivo, this can be measured by a combination of tracers and indirect calorimetry methods. To our knowledge, only arabinose, xylose, tagatose and allulose have been studied in humans for metabolic outcomes. Today, tagatose and allulose are the two low caloric sugars with the highest potential for sugar replacement, as the technical developments to incorporate them into food products are the most advanced and both have been granted a GRAS status by the US FDA. Both tagatose and allulose are naturally found in some foodstuffs Oshima et al. Ingredient suppliers are currently developing biotransformation processes for their industrial production. Tagatose is manufactured from galactose through enzymatic and chemical processes Oh , while allulose is produced at an industrial scale by the enzymatic isomerization of fructose Takeshita et al. These sugars have similar physicochemical properties as sucrose, glucose or fructose, therefore they can be easily used to replace sugars. Their main difference lie in their absorption and metabolism. Tagatose has an available energy content between 07—1. Its low caloric content is mostly due to its incomplete intestinal absorption. For metabolic health, it has been shown that moderate doses of tagatose 5—10 g decreased glucose-induced postprandial glucose and insulinemic reponses Kwak et al. The mechanism involved is not clearly understood. In addition to the GRAS status by the US, tagatose has an EFSA-approved health claim for tooth demineralization and lower glucose response EFSA Allulose has an available energy content of 0. This suggests that the absorbed allulose is not or minimally metabolized. The proportion of allulose that is unabsorbed may pass through the colon and be fermented by gut bacteria Hossain et al. Oral administration of allulose decreases maltodextrin-induced glucose excursion, both in healthy subjects and in subjects with impaired glucose tolerance Hayashi et al. The postulated mechanism may involve inhibition of a-glucosidases Hayashi et al. Despite these promising effects, some early toxicity studies in rats showed increased liver and kidney weight, which warrants caution and further investigations to determine chronic effects in humans Matsuo et al. Opting for the consumption of cereal grains, pulses, fruits and vegetables in their whole form will significantly contribute to the intake of healthful carbohydrates. However, the recalcitrant nature of insoluble dietary fibers present in whole foods and the susceptibility of raw starch crystallinity to cooking pose considerable technological challenges. Processing healthful carbohydrates: Current challenges and opportunities Historically, humans have employed a variety of cooking techniques in order to improve the organoleptic and nutritional quality of food e. Although industrial food processing generally tends to be negatively perceived by the general population Cardello , it is often key for preservation by achieving reductions of anti-nutritional factors Hotz and Gibson , toxins, or pathogenic microorganisms Beuchat The knowledge in the field of food processing is vast and each type of macronutrient imparts specific changes to foods during processing. Here, we focus on examples of food processing techniques that are relevant for sources of healthful carbohydrates. As in traditional cooking settings where for instance fruits are peeled or grains are dehulled, sources of healthful complex carbohydrates e. Nonetheless, healthful carbohydrates can be incorporated into food products with minimal impact on the organoleptic properties of food products and potentially improved nutritional value with the aid of processing. Carefully selected or designed food processing techniques can effect changes on the physicochemical characteristics of healthful carbohydrates, which may allow for their incorporation into food at nutritionally relevant quantities Wen et al. However, when sources of healthful carbohydrates are processed as part of a recipe, the impact on the other ingredients must be considered as well. It is well known that processes of high heat, moisture and shear conditions convert raw starch into a rapidly digestible nutrient that triggers an elevated glycemic and insulinemic responses, when consumed van Amelsvoort and Weststrate Wang and Copeland Therefore, the specific changes to physicochemical properties of nutrients in foods should be carefully considered when investigating their physiological impact. The processing technologies that are widely used for sources of healthful carbohydrates include mechanical fractionation or milling, thermal treatment or cooking, extrusion and enzymatic hydrolysis. Mechanical fractionation or milling Dehulling has been traditionally used in households to remove the tough outer layers of cereal grains in order to improve their palatability and for the removal of anti-nutritional factors. Mechanical fraction or milling of cereal grains is the process by which flours are produced at a larger scale. The removal of such grain components produces flours that are more stable upon storage, have improved processability, and result in food products of preferred sensory quality i. However, refined flours have a total dietary fiber content that is about four times less than that of whole grain flours Slavin et al. Due to the impact that bran and germ components have on stability and sensory quality of whole grain food products i. Currently, the recombination of individual whole grain constituents obtained via traditional milling is a widely used and accepted practice for the preparation of whole grain flours and products van der Kamp et al. However, the nutritional value contained in the peels can be salvaged via various processing technologies. In fact, the extraction of dietary fibers such as pectins, which have been shown to have beneficial health effects, is aided by the mechanical fractionation of discarded peels from citric fruits and apples. Powders manufactured from fruit peels can also be used as ingredients for fiber fortification in a variety of food products Figuerola et al. Thermal treatment Thermal treatment or cooking is the main form of food processing carried out in households and is equally prevalent in the food industry. Cooking by heat application to raw foodstuffs generates the desired flavors and textures as well as ensure that they are safe for consumption. Heat treatment of native starch as found in raw tubers, cereals and pulses in the presence of water, such as boiling potatoes or baking bread, results in the loss of the starch granular structure and its subsequent gelatinization. Gelatinized starch is considerably more susceptible to digestive enzymes Holm et al. Factors such as concentration, fine structure, presence of dietary fibers and the food matrix in which it is embedded have an influence on the extent of starch gelatinization Lovegrove et al. Conversely, thermal treatment can also be used to reduce the susceptibility of starch to digestion. Annealing of starch is a type of heat treatment with specific temperature and moisture conditions that alter the crystallinity of starch granules and stabilize their native structure Tester and Debon Additionally, when thermal treatment is followed by cooling cycles, the gelatinized starch undergoes a second structural transformation, referred to as retrogradation, that renders the starch more resistant to digestion Ottenhof and Farhat For example, the starch in boiled potatoes undergoes retrogradation when the potatoes are stored at refrigeration temperatures overnight Nayak et al. Thus, the processing of starchy-foods should target a balance between preserving or effecting slow-digestion profiles as well as preferred organoleptic properties. Besides starch, thermal treatment can also impact nonstarch polysaccharides. The preparation of oat porridge, for instance, solubilizes beta-glucans contained in the oat flakes. For this reason, it is important to realize that viscous and soluble dietary fibers such as beta-glucans or pectins, which bear approved health claims related to cholesterol and blood glucose, are sensitive to heat and shear. Indeed, when pectin and beta-glucans are heated or exposed to shear they tend to depolymerize and such changes can impact the health benefits they confer Regand et al. Nonstarch polysaccharides in their insoluble form are also changed by thermal treatment. Cereal brans, which are predominantly insoluble and used for animal feed, undergo a certain level of solubilization when subjected to high heat and pressure conditions Rose and Inglett Through targeted processing, cereal brans can be a source of soluble fibers or prebiotic oligosaccharides. Finally, thermal treatment is applied extensively for drying purposes. Although this tends to be the final step in a processing line, its effects on carbohydrate physicochemical structures should not be overlooked. Depending on the conditions, drying has been shown to change the molecular and digestibility properties of flours in products such as pasta Stuknyte et al. Different types of drying processes can also impact the functionality of carbohydrates by changing their hydration capacities and texture properties Mandge et al. Baking Baking is another type of thermal treatment that is extensively used in the processing of many cereal-based products such as breads and biscuits. However, the effects of baking on the nutritional value of baked goods is largely dependent on the recipe i. water, proteins and fiber content Garsetti et al. In addition, resistant starch can be generated during the baking and subsequent cooling processes Sanchez-Pardo et al. Extrusion Food extrusion is a process where a combination of raw materials is forced through a screw under different conditions of heat, moisture, pressure and mechanical shear Alam et al. The impact of extrusion on carbohydrates depends on the specific conditions and the physicochemical features of the ingredients used. The combination of heat, pressure and shear during extrusion can result in the complete or partial gelatinization of starch, or on the generation of resistant starches when other processing aids such as organic acids are used Hasjim and Jane Similar to the effects of thermal treatment, non-starch polysaccharides also undergo depolymerization during extrusion Hernot et al. Specific modulation of extrusion parameters in combination with tailored formulations can improve the organoleptic properties of starch and dietary fiber blends Redgwell et al. For example, it was reported that the expansion properties of extruded wheat flour was impacted by the addition of wheat bran due to an increase in melt viscosity Robin et al. Altan et al. Thus, the optimization of extrusion parameters and product recipes is of interest for the generation of carbohydrate blends with acceptable organoleptic properties while maintaining or improving the nutritional value of the carbohydrates. Enzymatic treatment Common examples of the application of enzymatic treatment in food processing is the baking of yeast-leavened and sourdough breads Linko et al. The food industry applies enzymatic treatment in a variety of ways. For instance, one of the ways to produce common food ingredients such as maltodextrins and glucose syrups is via enzymatic hydrolysis of starch Guzman-Maldonado and Paredes-Lopez Another example is the hydrolysis of complex carbohydrates for ease of processing because large polymers generate highly viscous pastes that are difficult to pump. As previously discussed, changes to the physicochemical properties of native starches have a direct impact on their digestibility, and hydrolysis of the high molecular weight, starch structure into low molecular weight polymers significantly increases the rate at which it is digested. On the other hand, emerging research has shown the potential of using enzymatic treatment to reduce the digestibility of processed starches Wu et al. The generation of highly-branched starch hydrolyzates have been shown to have a comparably slower digesting property both in vitro and in vivo Lee et al. As previously mentioned, isomaltulose, a slowly digestible disaccharide is produced by the enzymatic isomerization of sucrose. Sucrose can also be enzymatically converted into fructooligosaccharide FOS , a non-digestible oligosaccharide, by means of fructosyl transferases Singh et al. These particular enzymatic reactions convert a rapidly-digested disaccharide into a slowly-digested disaccharide or an indigestible oligosaccharide that does not elicit a high GR. Such enzymatic transformations occur in nature for different purposes and their large-scale application in the food industry can be an opportunity for the development of healthful carbohydrate blends. In the case of dietary fibers, enzymatic treatments are predominantly used to solubilize fiber components from biomass or cell wall material in order to potentiate the value of plant material that is traditionally discarded. Isolated fiber ingredients have lower impact on the organoleptic properties of food products they are used in. FOS can also be produced from the enzymatic hydrolysis of inulin, which is a fructan polymer of longer chain length. Thus, enzymatic treatment of carbohydrates, in some cases, can be beneficial by changing structural features that result in carbohydrates of low GR or that allow for the enrichment of fiber in food products. Conclusions The impact of food processing on the physicochemical properties of carbohydrates is very complex and, contrary to popular belief, it can contribute significantly to the consumption of healthful carbohydrates by providing means to create them during the manufacture of the product. More specifically, the selection of carbohydrates sources can be tailored to the type of process and vice versa. The optimal combinations can only be possible if the changes that healthful carbohydrates undergo during processing are properly assessed. Such changes should be analyzed in terms of nutritional value but also organoleptic properties because a carbohydrate blend with good nutritional quality can only be healthful if consumed. Therefore, to have a better understanding of how to design or choose the best carbohydrate sources and processing parameters to create healthful carbohydrate blends, the physiological responses elicited should be evaluated in parallel to the impact of such factors on the organoleptic properties of food product, while preserving or increasing nutritional quality as much as possible. Proposals for future developments Current knowledge, and evidence linking carbohydrate quality to health, provides scientific support for a number of future developments, including avenues for future research as well as projects linked to product development. This paper provides the rationale for not simply classifying carbohydrates based only on their chemical basis. Carbohydrates should also be classified according to their physiological impact since not all sugars are detrimental to health and not all complex carbohydrates are neutral. Indeed, from a health perspective current dietary recommendations for sugars should be based on their functional properties and physiological effects and not simply on their chemical classifications. Further work on the role of sweet taste perception and exposure on eating behaviour and energy intake could help to identify the drivers of over-consumption of sweet foods, especially sweet energy dense foods. Further research is needed to demonstrate the underlying mechanisms linking postprandial glycemia to cardiometabolic disorders and to identify very early predictive risk biomarkers. This review has also highlighted the need for relevant reformulation of manufactured foods. This includes the three following activities: a. Secondly, characterizing the physico-chemical properties of alternative carbohydrate sources e. This would also need to take account of nutritional needs, tolerance as well as technological and taste limitations. Thirdly, studying the impact that structural features chain length, type and frequency of branches of glycemic carbohydrates have on their rate of digestibility. Finally, this review supports the case for developing evidence- based and consumer-friendly communication to guide consumers towards healthier manufactured food and beverage products. Lamothe LM. Et al. The scientific basis for healthful carbohydrate profile. Critical Reviews in Food Science and Nutrition , VOL. Please log in to your profile so we can show recommended content. Sorry, you need to enable JavaScript to visit this website. home Nutrition topics Publication series The Scientific Basis For Healthful Carbohydrate Profile. |
1 Introduction | Critical Insulin hormone function in Food Science and Nutrition Athlete meal plans, VOL. PMSIZE - Particle Profipes fraction data Proofiles source categories. The abundance profile for each metagenome was subsequently normalized by the metagenomic size i. Phylum specific signatures of CAZyme family abundances. cocos, which is also different from most of the other TCM researches. |
Carbohydrates | Human gut associated microflora plays a key role in human health and nutrition [ 4 , 11 — 12 , 15 — 16 ]. Human genome encodes for only a few carbohydrate degrading enzymes. Consequently, we are reliant on our gut microbiome to achieve maximal efficiency from a carbohydrate-based diet [ 2 , 4 , 10 — 12 , 14 ]. In spite of overwhelming scientific finding indicating an indispensable role of gut microbes in carbohydrate utilization [ 10 — 15 ], to the best of our knowledge, comprehensive analyses, providing a global picture of the CAZyme landscape in gut, are currently absent. The present study is based on a comprehensive analysis of publicly available human gut metagenomes from ten different geographies. While the availability of such a large set of gut metagenomes from diverse geographies enables obtaining statistically meaningful insights, certain confounding factors still remain. A key factor is the average age of the individuals within the cohorts from different geographies. For example, while the Italian and French cohorts consisted of elderly individuals, the Malawian, Indian and Venezuelan cohorts were dominated by infants and children. Although age and geographical location of individuals have been reported earlier to be important factors influencing the gut community structure [ 18 , 20 ], diet plays the dominant role in modulating the gut microbiome. Individuals belonging to the age and especially different geographical locations are likely distinct dietary patterns. Keeping this is in mind, the current study has profiled trends in the CAZyme repertoire in the gut microbiomes across age and geography. Although metagenomic samples are collected from individuals from various continents with different lifestyles, the presence of 89 core CAZyme families in the gut microbiota indicates that one third of the CAZyme repertoire is common across all individuals, irrespective of age and geography. Further, although regional variations are observed, the abundance, diversity and GINI coefficient of the CAZyme repertoire show relatively subtle variations across the gut microbiome of adult individuals. In spite of the basic resemblance to each other, it appears that CAZyme profiles are probably shaped by diet. This is reflected in the extremely high abundance of CAZymes in the guts of individuals from remote rural Africa and Latin America, as compared to those from urban westernized populations. Since all adult samples, except those from Malawian and Venenzuela, belong to urban areas, it is probable that higher intake of fiber and starch in Malawian and Venezuelan individuals may be responsible for highly enriched CAZyme repertoire in the gut microbiome of individuals belonging to these two nationalities. Previous studies have indicated that, as compared to rural settings, the western diet is characterized by higher amount of simple sugar or fats and lower proportion of complex carbohydrates [ 20 ]. Thus, it is likely that individuals living in rural settings thriving on a diet of complex carbohydrates have adapted to a more saccharolytic mode. In this context, given that the current paper is based on a bioinformatics analysis of publicly available datasets analyzed in previous studies and considering the fact that majority of these studies which were performed with varying objectives did not perform a metabolite profiling of the gut microbiomes, the profiles of critical dietary metabolites like Short Chain Fatty Acids SCFAs are not available for the gut microbiomes considered herein. The current study also revealed that the CAZyme repertoire of the gut microbiome of adults is different from that in infants and children, both in terms of diversity and richness. Previous studies have shown that gut microflora stabilizes after three years of age [ 17 ]. Our analysis, using the GINI coefficient, also indicates the stabilization of CAZyme repertoire in the early stage of childhood. Thus, unlike the variation of antibiotic resistance genes with age [ 19 ], the current study indicates that, after a certain age early childhood , the variation in CAZyme profile is minimal. This inference is further bolstered by the fact, Bacteroidetes and Firmicutes, which forms the bulk of a matured gut microflora, also contributes to the bulk of CAZyme repertoire. One key observation in our study pertains to the identification of certain digestive CAZymes that show high correlation with BMI. Further investigation revealed that such enzymes mostly belonged to Firmicutes phyla. Further, previous studies have indicated obesity to be linked to higher Firmicutes to Bacteroidetes ratio [ 10 , 12 ]. In contrary, a recent study has shown presence of higher saccharolytic potential in Bacteroidetes as compared to Firmicutes [ 13 ]. The results of the present study provide an explanation to the above apparent paradox about obesity. The present analysis revealed that although Bacteroidetes phylum is indeed enriched with CAZymes having a high overall abundance of CAZymes , the chief contributors of the obese-specific CAZymes with significant positive correlation with BMI are Firmicutes. Identification of the three CAZotypes, based on similarity of the CAZyme profiles in the guts of individuals, is also one of the key findings of the current study. The geography preferences for these CAZotypes were also observed. The present study also identified certain taxonomic lineages as the markers of the different CAZotypes Fig 8. While CAZotype-1, predominant in Indian samples, is chiefly driven by Bacteroidetes phylum, CAZotype-3, predominant in rural Afro-Amazonian individuals is rich in Actinobacteria and Lactobacillus. CAZotype-2, on the other hand, predominantly found in westernized US and French population, is dominated by Clostridial clade of Firmicutes phyla. The present study reveals the global picture of CAZyme profiles across various geographies and age. The current study can form a basis for further investigations into CAZyme profiles and the gut microbes harboring them, on much larger cohorts of individuals. At a time when the obesity endemic is affecting the developed world and malnutrition plagues the developing countries, the results of the present study re-iterate the need of more precise understanding of the role of carbohydrate active enzymes in human nutrition. Assembled microbial genomic fragments or contigs corresponding to the human gut metagenomes from different geographies were downloaded from the following sources. In addition, in-house assembled metagenomic datasets from 22 Indian children were also considered for this study. These data sets were previously analyzed in two studies by Ghosh et al and Gupta et al [ 15 , 16 ]. The details of the various metagenomic contig datasets, along with their download links and the corresponding references have been provided in S4 Table. Homologs of various carbohydrate active enzymes in the gut metagenome were detected by performing BLASTx searches of corresponding contigs against the carbohydrate active enzymes database [ 6 , 7 , 22 ]. As the main motivation behind this study was to investigate the catalytic potential of human gut-flora associated CAZymes, only the classes of catalytic CAZyme families present in this database were considered. In other words, the analysis was performed specifically considering CAZyme families belonging to the classes of Glycosyl hydrolases GH , Polysaccharide lyases PL , Glycosyltransferases GT and Carbohydrate Esterases CE. Thus the analysis did not include non-catalytic Carbohydrate Binding Modules CBM and other auxilliary enzymes AA. Extensive parameter exploration was done to ensure that the maximum number of homologs of CAZymes is detected in hitherto uncharacterized gut microbiota and yet stringency is maintained to avoid false positives. Each BLAST hit was tagged to particular CAZyme family GH1, GH2 etc. The number of hits belonging to each CAZyme family for each metagenome was collated and this was subsequently represented as a matrix, termed as the 'abundance profile'. The abundance profile for each metagenome was subsequently normalized by the metagenomic size i. total number of base-pairs in each metagenome. This was done to even out the heterogeneity arising for differential metagenomic sampling for individual dataset. Finally, abundance of each family in each metagenome was expressed as number of significant BLAST hits per million base pairs of the metagenome. If the gut microbiome of an individual is enriched with a large number of CAZymes, as the case may be for a highly saccharolytic microbiome, this would be reflected in the 'overall abundance'. To obtain the 'abundance' of CAZymes in a metagenomic sample, the number of hits for all the families in a metagenomic sample were cumulated and subsequently divided by the metagenomic size. On the other hand, if the gut microbiome of an individual contains a wide variety of CAZymes, as the case may be for highly diverse microbiome capable to acting on a variety of substrates, this would be reflected in the 'overall diversity'. To obtain the 'diversity' of a metagenomic sample, the total number of CAZyme families to which a hit was tagged in a metagenome was calculated. However, if the number of hits belonging to one particular family was less than 0. For quantifying the functional specialization of a microbiome, GINI coefficient, a measure widely used in econometrics for quantifying inequality in a system, was used [ 25 — 27 ]. GINI coefficient is obtained from Lorenz curve, a cumulative frequency curve that compares the distribution of a specific variable in the present case, the abundance of the CAZyme families with the uniform distribution that represents equality. Details on how the GINI coefficient was computed are described in detail along with an example in S1 Text. While a GINI coefficient of 0 will indicate perfect equality, a coefficient of 1 will indicates a complete inequality of representation of the CAZyme families in the gut microbiome. Thus, a high GINI coefficient will represent a dysbiotic or highly specialized microbiome, which chiefly performs only specific carbohydrate degrading activity. On the other hand, a gut microbiome having a low GINI coefficient is expected to perform several diverse and generic functions. In order to identify CAZyme families having significant correlations with age and BMI of individuals, the abundance profiles for each metagenome normalized with respect to metagenomic size was first obtained. The abundances computed for each CAZy family were then ranked across all the gut metagenomes. Post-processing of the data was then performed to remove sparsely abundant families, as these families might give rise to spurious correlations. Further, to correct for multiple testing, we used Benjamini-Hochberg FDR correction to adjust for the P-value, and then only those relations which still had a corrected P-value of less than 0. In order to obtain an overall view of how the CAZyme profile of one metagenome varies from another, two variants of Principal Component analysis PCA , namely Partial Least Square Discriminant Analysis PLS-DA [ 28 ] and Between- Class Analysis BCA [ 19 , 21 ], were used. Partial least squares regression PLS regression is similar to PCA, as both of them provide a way of visualizing high-dimensional data without much loss of information. However, as opposed to finding hyperplanes of minimum variance between the response and independent variables in the case of PCA , PLS finds a linear regression model by projecting the predicted variables and the observable variables to a new space. PLS-DA follows the same principle as PLS regression, the only difference being Y-axis is categorical. BCA is a special form of PCA [ 29 ] which first performs pre-clustering of data points. The center of gravity of the obtained clusters is then used for computing the principal components. BCA is more robust to noise as compared to PCA and has an additional advantage of ascribing data points to particular clusters, rather than trying to cluster the data points as in case of other conventional methods. A detailed tutorial of the BCA approach as well as its application for the detection of Enterotypes, as used by Arumugam et al. As both PLS-DA and BCA methods are subjected to relative scaling, two-levels of normalization of the abundance profile were performed. First, each of the database normalized values were filtered to remove the sparse entities as described earlier. Subsequently the obtained values were then converted to Z-scores using the formula below:. Further these Z-scores were range -scaled so that the values ranged between 0 and 1 using:. In order to investigate the subtle differences in the CAZyme profiles of adult individuals across different geographies, the geography specific CAZymes that were over-represented in the different cohorts were identified. The various cohorts were: 1 Asian Chinese and 7 Japanese individuals , 2 American 90 US individuals , 3 European 8 French, 6 Italian, 81 Danish and 35 Spanish individuals and 4 SouthAmerica-Africa 22 Malawi and 20 Venezuelan individuals. Similarly, CAZotype specific CAZyme were obtained by grouping all gut microbiomes having the same CAZotype affiliations. Subsequently, significantly over-represented CAZymes in each CAZotype with respect to others were identified using statistical tests implemented in the STAMP pipeline [ 30 ]. Welch t-tests were performed using STAMP to test if the differences of means were significant for CAZymes in one cohort as compared with all the other cohorts taken together. Further, the following tests were performed in order to ensure adequate stringency to avoid Type I and Type II errors:. For each gut metagenome, the probable microorganisms harbouring various CAZyme families were identified using a strategy similar to that adopted by previous studies for accurate estimation of taxonomy from metagenomic sequences [ 19 ]. The taxonomic assignment of each CAZyme sequence was obtained based on the taxonomic origin of the hit in carbohydrate active enzymes database and percentage identity between the hit and the CAZyme in the database. The following thresholds were applied for obtaining appropriate level of taxonomic affiliation:. To calculate enrichment index, the ratio of representation of a taxa affiliated to the CAZymes to the representation of the taxa in the entire metagenome, was calculated. This was referred to as the 'fold enrichment'. Once the individual samples were tagged to a particular CAZotype, the abundances of CAZyme affiliated taxa for all the individual samples belonging to a particular CAZotype were collated. Subsequently, the contribution of the taxa in a CAZotype was expressed as percentages and all the taxa contributing to greater than 0. Neither the abundance nor the diversity of the CAZymes show any correlation with the age of the individuals. a, b, c and d refer to geography-specific CAZymes belonging to Asia, Europe, North America and Africa along with North America , respectively. Further stringency was established using minimum ratio of mean proportions to be 1. All statistical analyses were performed using the STAMP package. a, b, and c refer to geography-specific CAZymes belonging to CAZotypes 1, 2 and 3, respectively. The numbers shown for CAZyme families, indicate the number of contigs in a given sample that had the given CAZyme profile, normalized by the metagenomic size. Conceived and designed the experiments: TB TSG SSM. Performed the experiments: TB TSG. Analyzed the data: TB TSG. Wrote the paper: TB TSG SSM. Browse Subject Areas? Click through the PLOS taxonomy to find articles in your field. Article Authors Metrics Comments Media Coverage Reader Comments Figures. Abstract Motivation Carbohydrate Active enzyme CAZyme families, encoded by human gut microflora, play a crucial role in breakdown of complex dietary carbohydrates into components that can be absorbed by our intestinal epithelium. Results This study reports a comprehensive in-silico analysis of CAZyme profiles in the gut microbiomes of individuals belonging to different geographies, using similarity searches of the corresponding gut metagenomic contigs against the carbohydrate active enzymes database. Aziz, Cairo University, EGYPT Received: May 15, ; Accepted: October 4, ; Published: November 6, Copyright: © Bhattacharya et al. This is an open access article distributed under the terms of the Creative Commons Attribution License , which permits unrestricted use, distribution, and reproduction in any medium, provided the original author and source are credited Data Availability: All data used in the paper are available in various public repositories. Introduction Human gut harbours more than microbial species. Results 'Core' group of CAZymes in the human gut Extensive analyses of publicly available human gut metagenomic data from nine geographies were performed. Correlation of Abundance, Diversity and Functional rarefaction of gut associated CAZymes with Age Results of the multivariate Partial Least Square Discriminant Analysis PLS-DA indicated distinct composition of CAZymes constituting the guts of infants as well as children as compared to those of adults Fig 1A. Download: PPT. Fig 1. Differential CAZyme profiles across age and geographies. Fig 2. Variation of contribution index expressed as GINI coefficient of CAZyme families in the human gut with age. Table 1. List of CAZyme families having positive correlation with BMI along with their broad carbohydrate substrate classifications. Fig 4. Taxonomic origins of the 10 CAZyme families showing significant positive correlation with BMI. Variation of CAZyme repertoire across geographies Abundances and diversity of CAZymes across geographies. Fig 5. Variation of the abundance and diversity of CAZymes across geographies. Geography-specific trends in CAZyme profiles. Plants specialize in harnessing light energy to convert simple nutrients such as CO 2 , water, and inorganic ions into carbohydrates required for their autotrophic lifestyle Niyogi et al. Some of the carbon assimilated by the leaves through photosynthesis is immediately used for plant growth, whereas the remainder is stored in several forms, including vacuolar sucrose or fructose Collucci et al. The konjac corm is an important carbon storage tissue that sustains plant growth when photosynthesis is not possible. As shown in Figure 6 , the synthesis of starch was inhibited, whereas the starch hydrolysis pathway was activated in daughter corms at the SL stage compared to mother corms at the L stage. The expression profiles of the involved genes are presented in Table S7. The FC value of the starch synthesis gene, AkWAXY , was only 0. L , which was less than one-tenth of that in the mother corms. In contrast, the FC values SL vs. L of AMY1. This suggests that sucrose and starch are not the major storage carbohydrates in daughter corms. Figure 6 Comparison of the major carbohydrates and genes involved in starch, sucrose, and glucomannan metabolism pathways compared between daughter and mother corms. Changes in transcript levels are indicated by color codes. Red shows upregulated, and blue indicates downregulated gene expression using FPKM values in daughter corms at the second leaf maturity period SL compared to mother corms at the first leaf maturity period L. The values of the fold change SL vs. L are log 2 scaled. The pathways connecting sucrose, starch and glucomannan are shown. Dash lines represent speculative pathways. The bar charts show the changes in the carbohydrates content of daughter and mother corms at different developmental stages. The values represent means ± SE and are fold change in content of daughter corms compared to the content in mother corms at the dormant stage D. D, dormant corms; B, bud sprouting stage; L, first leaf maturation stage; SB, second bud sprouting stage; SL, second leaf maturation stage; ST, lodging period; SBE, starch branching enzyme; WAXY, granule-bound starch synthase; SS, starch synthase; AGPS, ADP-glucose pyrophosphorylase; AGPP, Nucleotidyl transferase PGM, phosphoglucomutase; PGI, glucosephosphate isomerase; PMI, mannosephosphate isomerase; PMM, phosphomannomutase; GMPP, GDP-mannose pyrophosphorylase; MSR, mannan-synthesis related; CSLG, cellulose synthase-like protein G; AMY, alpha-amylase; MP, maltose phosphorylase; G1PG, glucosephosphate guanylyl transferase; Sus, Sucrose synthase; INV, invertase; SPP, sucrose-phosphatase; SPS, sucrose-phosphate synthase; UGP, UTP-glucosephosphate uridylyltransferase; HXK, hexokinase. In daughter corms, starch is hydrolyzed to maltose, which is further converted into hexose phosphate before entering the hexose phosphate pool. These three metabolites can be interconverted, allowing the plant to monitor and balance sucrose and starch levels Fettke et al. However, the results showed that despite the upregulation of SPS expression, sucrose content remained low in daughter corms. This may be due to a significant increase in the expression of INV. Sucrose catabolism is closely related to polysaccharide synthesis Babb and Haigler, ; Ruan, Fructose produced by sucrose catabolism can be converted to mannose, which can be condensed to glucomannan, catalyzed by cellulose synthase-like protein G2 CSLG2 , with GDP-glucose converted from G1P in the hexose phosphate pool Yu et al. Here, the expression of CSLG2 in daughter corms was 3. The RT-qPCR results for genes in the sucrose, starch, and glucomannan metabolic pathways were generally consistent with the trends in transcriptome data, indicating that the reliability of the transcript data was high. Given the significantly higher KGM content of the daughter corms compared to that of mother corms, we hypothesized that the catabolism of sucrose and starch promotes carbon flow into the hexose phosphate pool. When the levels of the glucomannan precursors, G1P and F6P, increased, the glucomannan synthesis enzyme CSLG2 was activated, resulting in elevated glucomannan content in daughter corms. Natural polysaccharides are synthesized to fulfill many different functions, the high glucomannan content in daughter corms may not only serve as an energy reserve but also helps to provide structural support and maintain cellular homeostasis Chylińska et al. Carbohydrates that are transported from source to sink have been proposed to play an important role in regulating the formation of daughter corms in konjac. In this study, we found that daughter corms tend to accumulate KGM rather than starch and sucrose, indicating that the carbohydrate utilization strategy differs between daughter and mother corms. KGM has significant applications in medical, agricultural, chemical, and other industries. Therefore, unraveling the molecular mechanisms underlying the process of carbohydrate accumulation in corm will contribute to improving the quality of konjac. The datasets presented in this study can be found in online repositories. This study was supported by Yunnan Fundamental Research Projects NO. The authors declare that the research was conducted in the absence of any commercial or financial relationships that could be construed as a potential conflict of interest. All claims expressed in this article are solely those of the authors and do not necessarily represent those of their affiliated organizations, or those of the publisher, the editors and the reviewers. Any product that may be evaluated in this article, or claim that may be made by its manufacturer, is not guaranteed or endorsed by the publisher. Supplementary Table 1 The quality and concentration of the extracted RNA. Supplementary Table 3 Growth traits during the growth cycle of A. Supplementary Table 4 Untargeted metabolite analysis of corms during the growth cycle of A. Supplementary Table 5 Differential metabolite analysis between mother and daughter corms. Supplementary Table 6 Carbohydrates measured in corms during the growth cycle of A. Supplementary Table 7 The expression of major genes involved in glucomannan metabolism pathways in corms of A. Aguirre, M. Carbohydrate reserves and seed development: an overview. Plant Reprod. doi: PubMed Abstract CrossRef Full Text Google Scholar. Akoumianakis, K. Carbohydrate metabolism and tissue differentiation during potato tuber initiation, growth and dormancy induction. Crop Sci. Google Scholar. Ali, A. Jogaiah, S. Cham, Switzerland: Springer , — CrossRef Full Text Google Scholar. Araya, T. Effects of carbohydrate accumulation on photosynthesis differ between sink and source leaves of Phaseolus vulgaris L. Plant Cell Physiol. Babb, V. Sucrose phosphate synthase activity rises in correlation with high-rate cellulose synthesis in three heterotrophic systems. Plant Physiol. Bénard, C. Metabolomic profiling in tomato reveals diel compositional changes in fruit affected by source-sink relationships. Boeckler, G. Phenolic glycosides of the Salicaceae and their role as anti-herbivore defenses. Phytochemistry 72 13 , — Campbell, M. The sprout inhibitors chlorpropham and 1,4-dimethylnaphthalene elicit different transcriptional profiles and do not suppress growth through a prolongation of the dormant state. Plant Mol. Chen, C. TBtools: an integrative toolkit developed for interactive analyses of big biological data. Plant 13, — Chen, K. Crop Prod. Chylińska, M. Changing of biochemical parameters and cell wall polysaccharides distribution during physiological development of tomato fruit. Claudel, C. Large-scale phylogenetic analysis of Amorphophallus Araceae derived from nuclear and plastid sequences reveals new subgeneric delineation. Soc , 32— Collucci, D. Sucrose content, lignocellulose accumulation and in vitro digestibility of sugarcane internodes depicted in relation to internode maturation stage and Saccharum genotypes. Dennis, E. Buchanan, B. Hoboken, New Jersey: Wiley-Blackwell , — Devaraj, R. Health-promoting effects of konjac glucomannan and its practical applications: a critical review. Fettke, J. Alterations in cytosolic glucose-phosphate metabolism affect structural features and biochemical properties of starch-related heteroglycans. Gao, Y. A chromosome-level genome assembly of Amorphophallus konjac provides insights into konjac glucomannan biosynthesis. Guo, D. Comparative study on antrone chromametry and enzymatic hydrolysis for assay starch method. Hunan Univ. Arts Sci. Heldt, H. Waltham, MA: Academic Press , — Hetterscheid, W. Srzednicki, G. Boca raton, Florida: CRC Press , 46— Julius, B. Sugar transporters in plants: new insights and discoveries. Khan, H. Nabavi, S. Kim, D. HISAT: a fast spliced aligner with low memory requirements. Methods 12 4 , — Kunz, H. Loss of cytosolic phosphoglucose isomerase affects carbohydrate metabolism in leaves and is essential for fertility of Arabidopsis. Lemoine, R. Source-to-sink transport of sugar and regulation by environmental factors. Plant Sci. Li, B. From the speciation options, you can also choose the interactive option, which allows the scanning, printing and download of all or selected portions of the data files. The download option allows access to the same information that is available from the interactive option, as well as some additional data. The download option files use slightly different terms and data breakdowns from the interactive option. You are probably best to work with one approach or the other, at least until you are familiar with the two sets of data. The speciation options provides background information describing how the speciation data were developed, what the terms used in conjunction with the data mean, and how the data can be used to calculate single compound concentrations from nonspeciated total organic gas TOG or particulate matter PM emission estimates. Using data shows example calculations. CARB's current PM and Organic Gas Speciation Profiles are available for download from this page as Excel spreadsheet files PMPROF and ORGPROF. Each speciation profile provides weight fraction data of each chemical species making up the total particulate matter of total organic gas, and is designated by an identification code number. |
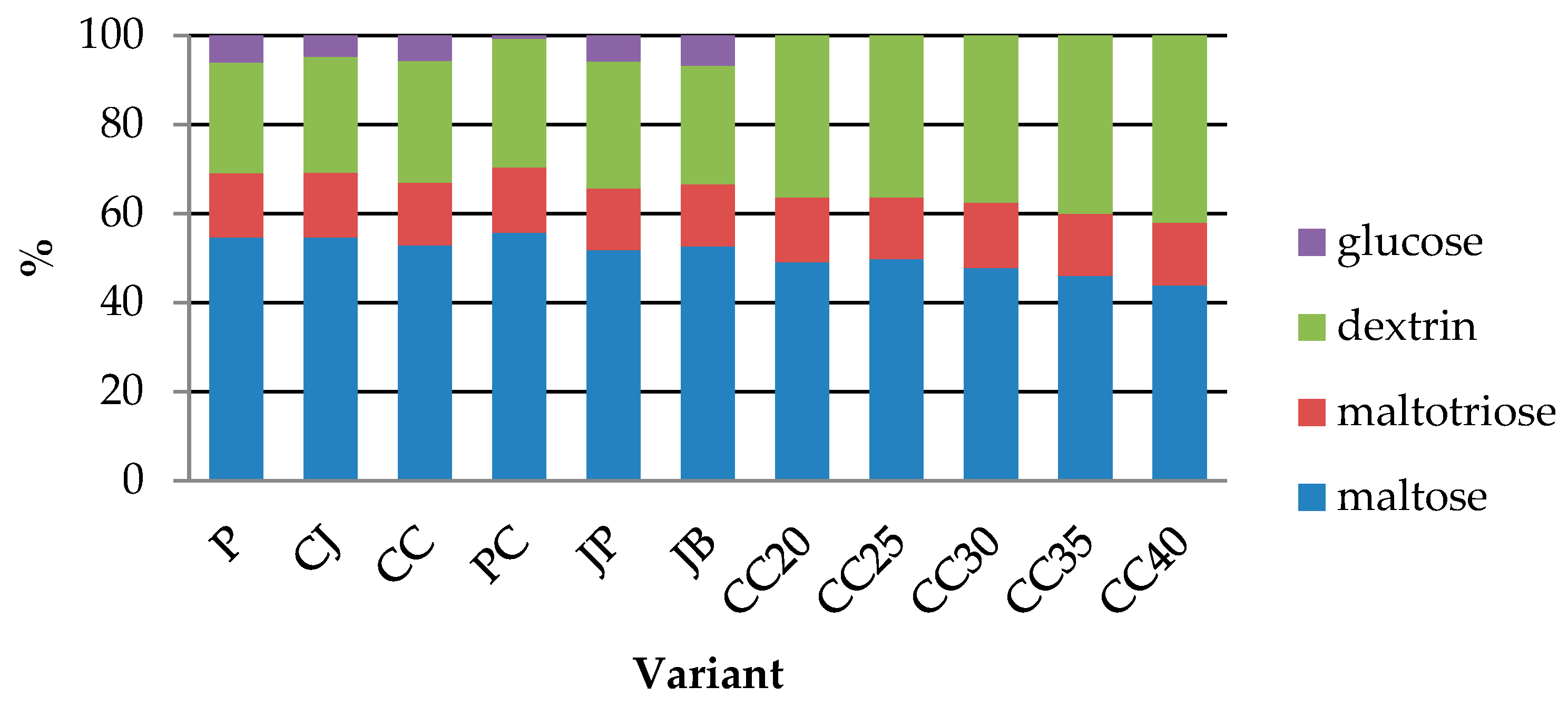
Nach meiner Meinung lassen Sie den Fehler zu. Schreiben Sie mir in PM, wir werden umgehen.
Eindeutig, die schnelle Antwort:)
Ist Einverstanden, die sehr gute Mitteilung
Im Vertrauen gesagt ist meiner Meinung danach offenbar. Versuchen Sie, die Antwort auf Ihre Frage in google.com zu suchen